Artificial Intelligence (AI) Engineer vs. Data Scientist
Artificial Intelligence (AI) Engineer vs. Data Scientist
Artificial Intelligence (AI) Engineer vs. Data Scientist
An architect’s spoken ideas for a new building move into reality. An image recognition system spots a rare cancer physicians can’t yet detect. Shoppers stroll through the metaverse, trying out products without leaving their armchairs. Artificial intelligence (AI) and data science applications are booming across multiple industries — increasing profits, optimizing decision-making, and offering ways to improve lives.
These developments stem from the work of AI engineers and data scientists, professionals with technology expertise, business sense, and ethical awareness. An advanced education, often boosted by a specialized certification, equips these sought-after professionals with the in-depth understanding and skills they need to help tackle challenges as tech hurtles into the future.
In particular, AI engineers and data scientists play a huge role in business, priming organizations to achieve their goals by influencing product development, guiding marketing efforts, and supporting data-driven decision-making.
When comparing AI engineer vs. data scientist roles, it’s clear their tasks and responsibilities dovetail in many ways. Yet the focus of their work is significantly different. Understanding the distinctions between these related roles can help people passionate about diving into the future of tech decide which career path to follow.
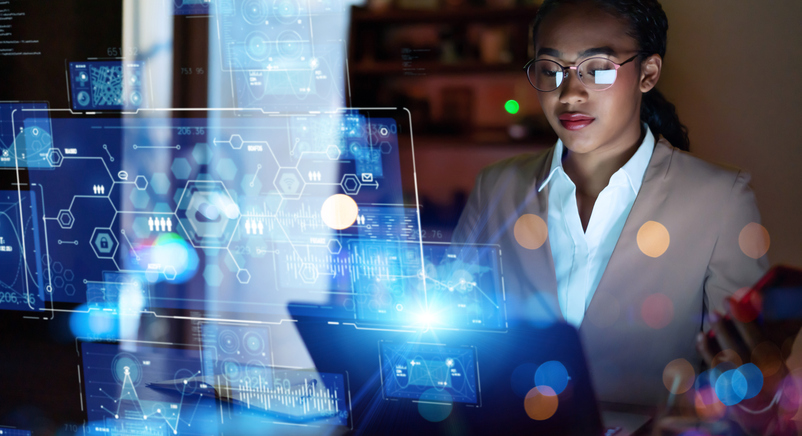
What Does an AI Engineer Do?
AI engineers train computers to process large amounts of data and find patterns in that data, according to SAS. The goal is to get machines to do tasks or solve problems. AI engineers help machines learn from situations, adapt to new information, and perform actions.
The main task of an AI engineer is to implement AI models using programming algorithms (rules or processes). In basic terms, an AI engineer applies insights from data.
Related tasks of an AI engineer include:
- Managing the AI product development process
- Developing infrastructure for data transformation and ingestion
- Automating infrastructure for the data science team
- Communicating with product managers and organizational stakeholders
- Transforming machine learning (ML) models into application programming interfaces (APIs), software that lets different applications talk to each other.
AI Engineering and Business
In businesses, AI engineers offer stakeholders vital information. “Because of its ability to handle large amounts of data and forecast outcomes, AI has become essential for strategic decision-making,” according to Forbes. While humans supply the gut feeling and common sense, AI-engineered systems leverage predictive analytics and other methods to suggest options decision-makers can choose to act on.
Other ways AI engineers help businesses grow and innovate, a process called enterprise AI, include:
- Automating repetitive business processes
- Standardizing data
- Analyzing customer feedback
- Providing precise data for customer relationship management
Organizations apply AI models in fields including:
- Healthcare: Healthcare facilities use AI to automate labor-intensive processes, such as those involved in drug discovery, to save time and money.
- Finance: Banks use AI models to monitor patterns of consumer behavior and detect suspicious activity on credit cards.
- Manufacturing: Factories use AI to make the manufacturing process safer and less costly by predicting maintenance issues, reimagining supply chains, and integrating with tech systems.
Trends in AI Engineering
Employer demand for AI engineering professionals in business is surging, and the AI engineering field is taking off in multiple directions. Trends for AI’s future include:
- Advancements in virtual and augmented reality
- Focus on AI in cybersecurity
- Focus on AI-controlled tech, such as robotics, drones, and driverless vehicles
- Emphasis on ethics and removing cultural biases from data
- Development of voice and language applications
What Does a Data Scientist Do?
Data scientists interpret the patterns they find in large volumes of information and offer advice to stakeholders based on their findings. These respected experts typically oversee the collection, storage, management, protection, and analysis of business data.
A primary responsibility of a data scientist is leveraging data to support an organization’s decision-making process. In doing so, they must know how to:
- Use programming languages
- Create data management software
- Build hardware and network systems
- Detect data security vulnerabilities
- Test and improve network functionality
Data Science and Business
Businesses rely on accurate data. Data scientists help businesses interpret data drawn from customers’ engagement with their products or services, and then use those interpretations to guide the creation of enjoyable, personalized encounters that encourage customers to spend money. The ability to monetize data is one key reason data scientists are in high demand.
The data collection that drives a company’s customer experience strategy must be precise — otherwise it won’t achieve results. Cutting-edge data collection tools that data scientists use to support accurate data-driven customer experience strategies include:
- SurveySparrow
- Fulcrum
- Teamscope
- Kobo Toolbox
- Magpi
- Jotform
Data scientists perform other valuable tasks for businesses as well, including:
- Analyzing data with an eye for improving business operations
- Weighing the success of recent business initiatives
- Automating data collection and storage
- Searching databases for specific purposes, such as determining employee retention rates or identifying market trends
- Reporting on their findings to upper management using data visualization tools
Trends in Data Science
A top trend in data science is the democratization of data, which refers to efforts to give more stakeholders access to understandable and easily accessible information to use for decision-making.
The democratization of data will result in swifter decision-making, more efficient teams, and, potentially, an edge on the competition, according to Adobe Cloud Experience. The key to accomplishing data democratization is automated machine learning (autoML).
AutoML applications go beyond business. “Developers of autoML solutions seek to create tools and platforms that can be used by anyone to create their own ML apps,” according to Bernard Marr in Forbes. “In particular, it’s aimed at subject matter experts whose specialized expertise and insights make them ideally placed to develop solutions to the most pressing problems in their particular fields but who often lack the coding knowledge needed to apply AI to those problems.”
AutoML allows people to explore ideas without barriers.
AI Engineer vs. Data Scientist: Comparison
AI engineers and data scientists have closely related but distinct roles. Each can be vital to a business. A comparison of AI engineers (sometimes known as machine learning engineers) vs. data scientists yields insight into the value of each role to an organization. It also reveals how the roles work together to benefit organizations.
Which Came First?
AI engineering is an outgrowth of data science. AI engineers need the information generated by data scientists through analytics to create powerful AI models and applications.
Marr expresses the relationship like this: “Broadly speaking, [data science] has enabled the emergence of machine learning (ML) as a way of working towards what we refer to as artificial intelligence (AI), a field of technology that’s rapidly transforming the way we work and live.”
The Data Pipeline
AI engineers and data scientists both work with the data pipeline. Data scientists collect data, store it, and keep it secure. They also prepare it by cleaning and shaping raw data into usable form. Then they use that information to make logical inferences that can be applied to business operations and goals.
In turn, AI engineers automate the interpretation of ever larger volumes of data. This creation of a decision-making process that emulates human thinking is called AI modeling. “These models use various types of algorithms, such as linear or logistic regression, to recognize patterns in the data and draw conclusions in a manner that emulates human expertise,” according to Intel.
While AI engineers and data scientists use data in similar ways, the work of AI engineers differs from that of data scientists in that it strives to use AI modeling to teach machines (software applications) to make decisions faster than humans ever could.
A Shared Focus on Ethics and Safety
AI engineers and data scientists share a focus on ensuring ethical business practices guide the use of technology. As tech has pervaded mainstream culture and crept into every corner of modern life, it has brought the flaws of the humans who created it to light: Facial recognition software has shown racial bias; synthetic data has been used for deep fakes, such as videos showing real people in situations that never happened; analytics have failed to predict crime.
Safety issues have risen to the forefront, too. Many tech safety issues revolve around a machine’s lack of the common sense that humans take for granted. For example, “In one study, the strategic placement of a few small stickers on a stop sign made a computer vision system see it as a speed-limit sign,” according to Matthew Hutson in The New Yorker. That scrap of misleading data could make all the difference in saving a life.
In September 2022, GM recalled the software used in its self-driving Cruise cars in San Francisco when one of them made an unprotected left turn into the path of an oncoming car, injuring two people. The crash occurred because the software made a wrong prediction about what the oncoming car would do.
“Progress in AI not only raises the stakes on ethical issues associated with its application, it also helps bring to light issues already extant in society,” James Manyika observes in Daedalus. AI engineers and data scientists can serve as ethical custodians of tech innovation. They can strive to ensure discrimination against marginalized groups, violation of the privacy of individuals through data breaches, and hazards brought about by faulty software predictions will not violate rights or cause harm.
Tech Skills
AI engineers and data scientists share a similar tech skill set. For example, each role requires knowledge of linear algebra, statistics, and programming.
However, a tech skill specifically associated with AI engineers is the ability to work with neural network architecture. Crucial to machine learning applications, artificial neural networks get their name from the biological neural networks they resemble. Basically, a neural network is a computing system made up of connected units that mimics the function of the brain.
AI engineers use neural network architecture in machine learning applications such as robotics, facial recognition, and medical diagnostics.
Salary
Professionals in both roles are highly compensated. However, AI engineers have higher salaries, on average, than data scientists.
As of September 2022, the median annual salary for a data scientist was around $98,000, according to PayScale, with experienced data scientists earning $137,000 on average.
As of September 2022, the median annual salary for an AI engineer was around $132,000, with experienced AI engineers earning $159,000 on average, according to PayScale.
Build a Strong Business Future Through Technology
In today’s global marketplace, technology and business are inextricably linked. AI engineers and data scientists are responsible for steering businesses through technical and ethical challenges to provide their customers and clients with an enjoyable, safe, and productive future, thanks to the generative solutions these complementary roles contribute.
If you feel called to participate in shaping world-changing innovations by applying your technical skills, ethical mindset, and business decision-making savvy to a rewarding and challenging career, learn more about Maryville University’s online Master of Science in Artificial Intelligence degree and AI certificates.
If you choose to complete both the Fundamentals of Artificial Intelligence and Advanced Artificial Intelligence certificate programs, you can apply the certificates toward a future online master’s in AI. Start building a future of unlimited possibility with training in AI.
Recommended Reading
Building Skills for the Future of Machine Learning
The Future of Artificial Intelligence in Work and Everyday Life
Sources
Adobe Experience Cloud, “Why Data Democratization Is Crucial to Your Business.”
Bernard Marr & Co., “Does Synthetic Data Hold the Secret to Artificial Intelligence?”
Daedalus, “Getting AI Right: Introductory Notes on AI and Society”
Forbes, “The Five Biggest Data Science Trends in 2022”
Forbes, “Here’s How AI Optimizes Executive-Level Decision-Making in Mega-Corporations”
Forbes, “Six AI Trends to Watch in 2022”
Indeed, Data Scientist Job Description: Top Duties and Qualifications
Indeed, Everything You Need to Know About the Role of an AI Engineer
The New Yorker, “Can Computers Learn Common Sense?”
Payscale, Average Data Scientist Salary
Payscale, Average Machine Learning Engineer with Artificial Intelligence (AI) Skills Salary
SAS, “Artificial Intelligence: What It Is and Why It Matters”
SAS, “Meet the Data Scientist: Alex Herrington”
SAS, “What Is a Data Scientist?”
Simplilearn, “Top 5 Jobs in AI and Key Skills Needed to Help You Land One”
Wired, “GM’s Cruise Recalls Self-Driving Software Involved in June Crash”
Be Brave
Bring us your ambition and we’ll guide you along a personalized path to a quality education that’s designed to change your life.