Predictive Analytics in Insurance: Types, Tools, and the Future
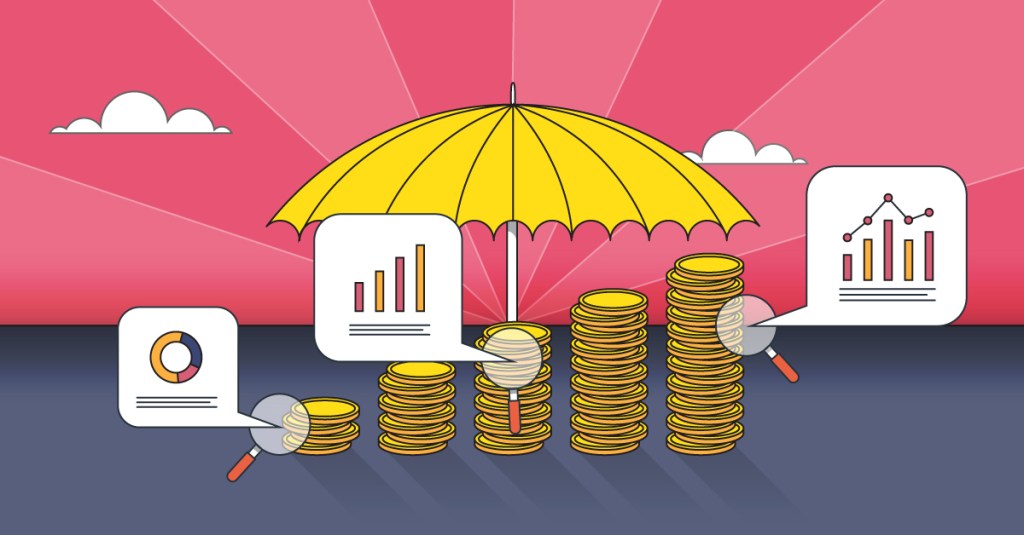
Tables of Contents
- What Is Predictive Analytics?
- Predictive Analytics – Life Insurance Industry
- Predictive Analytics – Health Insurance Industry
- Predictive Analytics Tools for the Insurance Industry
- The Future of Predictive Analytics Use in the Insurance Industry
- Delivering the Benefits of Big Data to the Insurance Industry
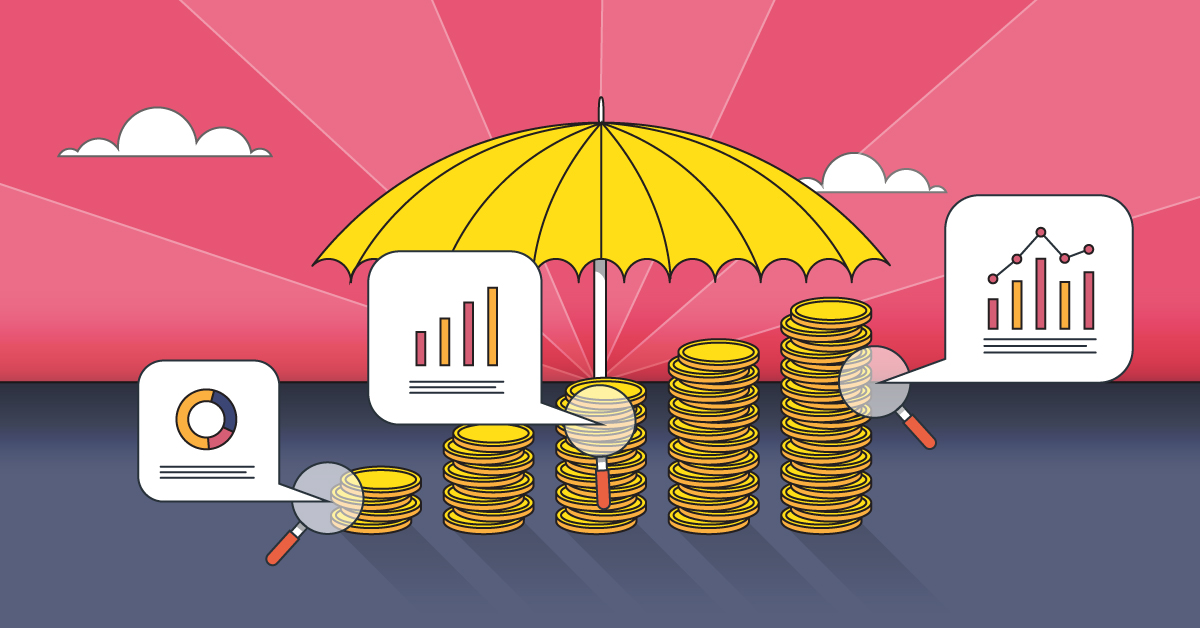
The insurance industry is based on a simple equation:
Better data = more accurate risk calculations = higher profits
In the age of big data and artificial intelligence (AI), insurance companies compete to have the highest-quality data and topflight analytics tools to convert the data into business intelligence. The state of the art in data analytics is predictive analytics, which SAS defines as the use of data, statistical algorithms, and machine learning techniques to forecast future outcomes based on historical data.
Predictive analytics is reshaping the insurance industry, which has relied on data from its inception centuries ago. This reliance on data analysis makes insurance uniquely suited to the use of predictive analytics. For example, a survey conducted by Willis Towers Watson found that life insurers who use predictive analytics reported a 67% reduction in expenses and a 60% increase in sales.
The impact of predictive analytics on the insurance industry is so profound that Towards Data Science forecasts the rise of “InsurTechs,” which are small, entrepreneurial companies with experience in applying data, AI, and mobile applications. These firms can pose a serious threat to established insurers that fail to “reinvent and modernize the applications that have been the source of their competitive advantage.”
What Is Predictive Analytics?
Investopedia answers the question, What is predictive analytics? as the process of identifying patterns in data to determine whether those patterns are likely to recur. Businesses and investors adjust their placement of resources to take advantage of future events based on the likelihood of past patterns repeating.
Predictive Analytics Definition for the Insurance Industry
From the perspective of the insurance industry, predictive analytics is the most versatile of AI applications currently available to solve business problems, according to AI research firm Emerj. While actuaries have always applied mathematical models to predict the likelihood of property loss and damage, insurance firms now see data analytics as a way to maximize the value of their data investments.
Insurers see prescriptive analytics as a way to improve customer experience while simultaneously reducing the time and cost of claims handling and eliminating fraud, according to Information Age. In addition, insurance firms are integrating external data sources with their own data stores to gain more insight into claimants and injured parties. However, they face challenges in combining identity verification, social media, and other external data with internal systems.
How Insurance Companies Assess Risk, Set Rates
Insurance premiums are the rates insured parties pay for the coverage they receive. Insurance companies employ actuaries to calculate the risk of insurance policies covering life, property, liability, and other kinds of insurance. The level of risk that the insurance company must assume determines the price of the insured party’s premium.
Actuaries rely on risk analysis software that applies statistical and mathematical models to demographic data about the insured party combined with a variety of data from external sources to calculate the likelihood of a death, sickness, injury, disability, or property loss, as The Balance explains. Based on the recommendations of actuaries, insurance companies invest the premiums, so they’re assured to have sufficient funds to pay out any potential claims.
How Predictive Analytics Improves Risk Model Accuracy
Information technology (IT) services firm LatentView Analytics describes the three steps involved in insurance underwriting:
- Collect relevant client information, including credit history, medical history, and driving record.
- Analyze the data to devise a risk score for the insured party.
- Apply predefined underwriting guidelines to accept or decline the application for the policy, and if accepted, calculate the client’s premium.
Predictive analytics and other AI technologies improve the accuracy of the risk models used by insurers by automating the process of adjusting data models, which saves actuaries a great deal of time and effort. Use of data models based on predictive analytics allows underwriters to make more accurate predictions about a client’s risk profile.
Underwriters gain “cognitive insight” to identify elements relevant to risk evaluations that traditional modeling methods miss. Much of this data is unstructured, such as emails, geospatial location, video, images, and data from smartphones and social media. Data sources include credit services, government agencies, financial services, and other third-party vendors.
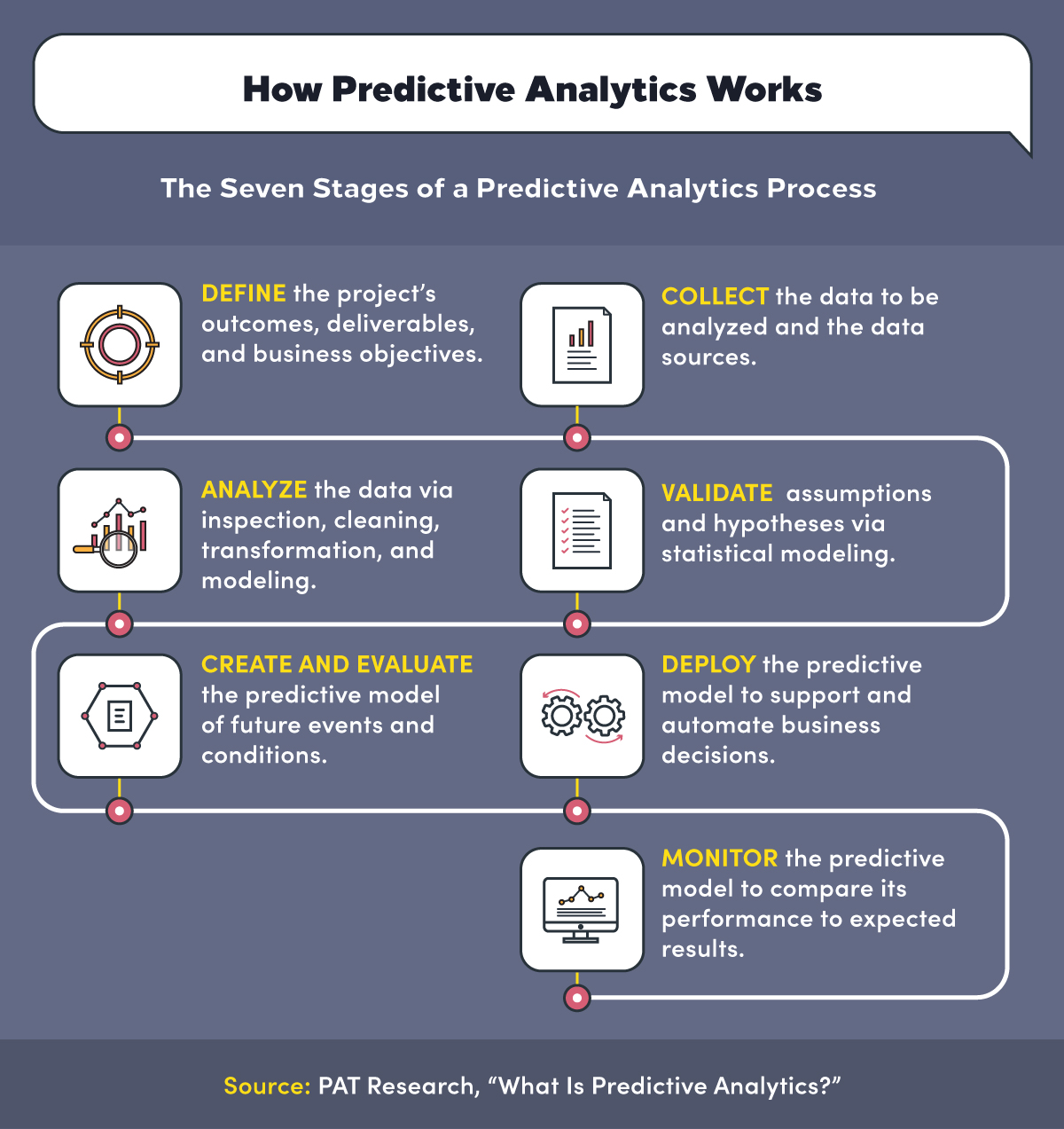
How Consumers Benefit from Insurers’ Use of Predictive Analytics
A large portion of the world’s population remains uninsured because they can’t afford the premiums required to be covered. The International Actuarial Association explains that inclusive insurance, which includes microinsurance, is intended to bridge the gap in coverage by making policies more affordable.
Developing economies lack a sufficient supply of actuaries, so the industry relies increasingly on “proportionate actuarial services” that are based on the level of risk and availability of risk mitigation. Predictive analytics tools are seen as a way to price insurance given a limited range of factors. Nonexperts can use the tools to project required reserves to cover a five-year policy, for example, by providing an automated risk profile and liability structure.
GoodData lists four ways insurers and their customers are benefiting from the use of AI-based analytics:
- The increased availability of potentially relevant data must be matched by modeling that separates high-quality data from low-quality data. By increasing the amount of quality data available, the model’s results will be more accurate.
- Predictive models score underwriting submissions to identify traits such as “broker sincerity” and “projected loss ratio for this class.” AI-based ranking systems help underwriters calculate the optimal course by connecting insight with action.
- By reducing the amount of guesswork entailed in decisions, AI-based models improve the accuracy and consistency of employees’ policy work. For example, adjusters with limited experience can be guided by the system to avoid overcharging a client for a policy or undercompensating for a claim.
- Much of the overhead insurers pay can be eliminated by automating fraud detection via AI-based pattern recognition. Insurers are able to pass much of the savings they realize to consumers by offering them lower premiums.
How Are Predictive Analytics Used in the Life Insurance Industry?
Predictive analytics is a natural extension of the methods insurers have used for several hundred years to calculate risk and set premiums for their policies. The manual underwriting methods that life insurers relied on in the past are time-consuming and expensive, as Oklahoma State University researcher Sai Gopi Krishna Govindarajula explains.
Predictive analytics in life insurance streamlines the underwriting process and improves risk assessment, which increases insurers’ profitability and customer retention rates. For example, use of predictive analytics combined with genetic profiling can help life insurance firms distinguish risk related to unhealthy lifestyles from risks associated with genetic factors that are beyond the insured party’s control, and then adjust the policy accordingly.
How Life Insurance Companies Benefit from Predictive Analytics
The Willis Towers Watson Life Predictive Analytics Survey Report from September 2018 shows the tremendous impact predictive analytics has already had on the life insurance industry. Four factors were rated highly important by life insurance companies in the drive to adopt predictive analytics:
- Competitive pressures in product development and pricing (cited by 78% of respondents)
- Customer relationship management (67%)
- Earnings and profitability pressures (64%)
- Technology innovation (60%)
The survey identified three areas in which predictive analytics has had the greatest impact on life insurers’ performance:
- Reduction in issue and underwriting expenses. 17% reported a strong positive impact, and 50% reported a somewhat positive impact.
- Significant increase in sales. 17% reported a strong positive impact, and 43% reported a somewhat positive impact.
- Increase in profitability. 13% reported a strong positive impact, and 47% reported a somewhat positive impact.
Application of Predictive Analytics by Life Insurance Underwriters
The expanded use of predictive analytics by life insurers can be applied to four specific functions:
- Pricing and rate-setting use is forecast to increase from 31% to 56% in two years for group life, and from 18% to 55% for individual life.
- Underwriting use may increase from 52% to 92% in two years for individual life.
- Mortality and morbidity risk use may increase from 19% to 56% in two years for group life, and from 23% to 75% for individual life.
- Claim management use may increase from 37% to 87% in two years for group life, and from 10% to 40% for individual life.
The Milliman IntelliScript predictive analytics-based tool generates a risk score for future mortality based on information from a person’s prescription drug history. Optimum Re, a company that provides technical services to the insurance industry, applied the Milliman IntelliScript algorithm to more than 25 million insurance applications. The analysis found that Milliman IntelliScript was a “good predictor of future mortality” and could be used to “replace traditional underwriting requirements.”
Statistics on Use of Predictive Analytics in Life Insurance
Intensifying competition and raising customer expectations are driving investment in predictive analytics in life insurance. Companies offering individual life insurance and group life insurance are expected to lead adoption of predictive analytics in the industry, according to the Willis Towers Watson survey.
- Current use of predictive analytics to calculate individual life policies (and expected percentage in two years):
- 70% of large carriers (increasing to 90% in two years)
- 50% of midsize carriers (75%)
- 54% of small carriers (89%)
- Current use of predictive analytics to determine group life policies (and expected percentage in two years):
- 71% of large carriers (increasing to 100% in two years)
- 67% of midsize carriers (100%)
- 23% of small carriers (46%)
- Current use of predictive analytics to calculate individual health policies (and expected percentage in two years):
- 40% of large carriers (increasing to 80% in two years)
- 20% of midsize carriers (40%)
- 15% of small carriers (38%)
The accuracy of predictive analytics methods depends on the availability and conditioning of reliable data to apply to the models. The Willis Towers Watson survey identified the most common sources of data for life insurers using predictive analytics as of September 2018 (and planned to use in two years):
- Internal customer data: 55% used as of September 2018 (82% planned to use in two years)
- Customer interactions and surveys: 55% (73%)
- Clickstream data: 18% (45%)
- Social media: 13% (35%)
- Web scraping: 11% (29%)
- Wearables 6% (38%)
The Challenges of Using Predictive Analytics in Life Insurance
Despite the potential of predictive analytics to improve life insurers’ operations and profitability, the industry faces formidable challenges in realizing the technology’s benefits. Principal among the obstacles to widespread use of predictive analytics by the industry is developing the infrastructure to accommodate the massive amounts of data required to run predictive analytics models.
The Willis Towers Watson survey found that 82% of large life insurers and 50% of midsize and small carriers were using or exploring the option of using cloud-based environments for their big data needs as of September 2018. Similarly, 45% of large life insurance carriers, 50% of midsize carriers, and 29% of small carriers were either using or exploring the option of using the Apache Hadoop framework for managing big data.
Another obstacle to widespread adoption of predictive analytics by life insurers is helping business stakeholders understand and act on the modeling results. Only 13% of the insurers surveyed believed the models were well understood or very well understood by people outside of data science and actuarial areas, and 40% stated that widespread understanding is very limited or nonexistent.
Resources for Predictive Analytics in Life Insurance
- Deloitte, 5 Ways to Sell More Life Insurance: This report describes how predictive analytics can stem the sharp decline in life insurance policies over the past 20 years.
- Canadian Institute of Actuaries, The Use of Predictive Analytics in the Canadian Life Insurance Industry: This study identifies several trends that impact the application of the technology.
- TechHQ, How Predictive Analytics Is Closing the Life Insurance Gap: This article examines the effect InsurTech firms have had on the industry.
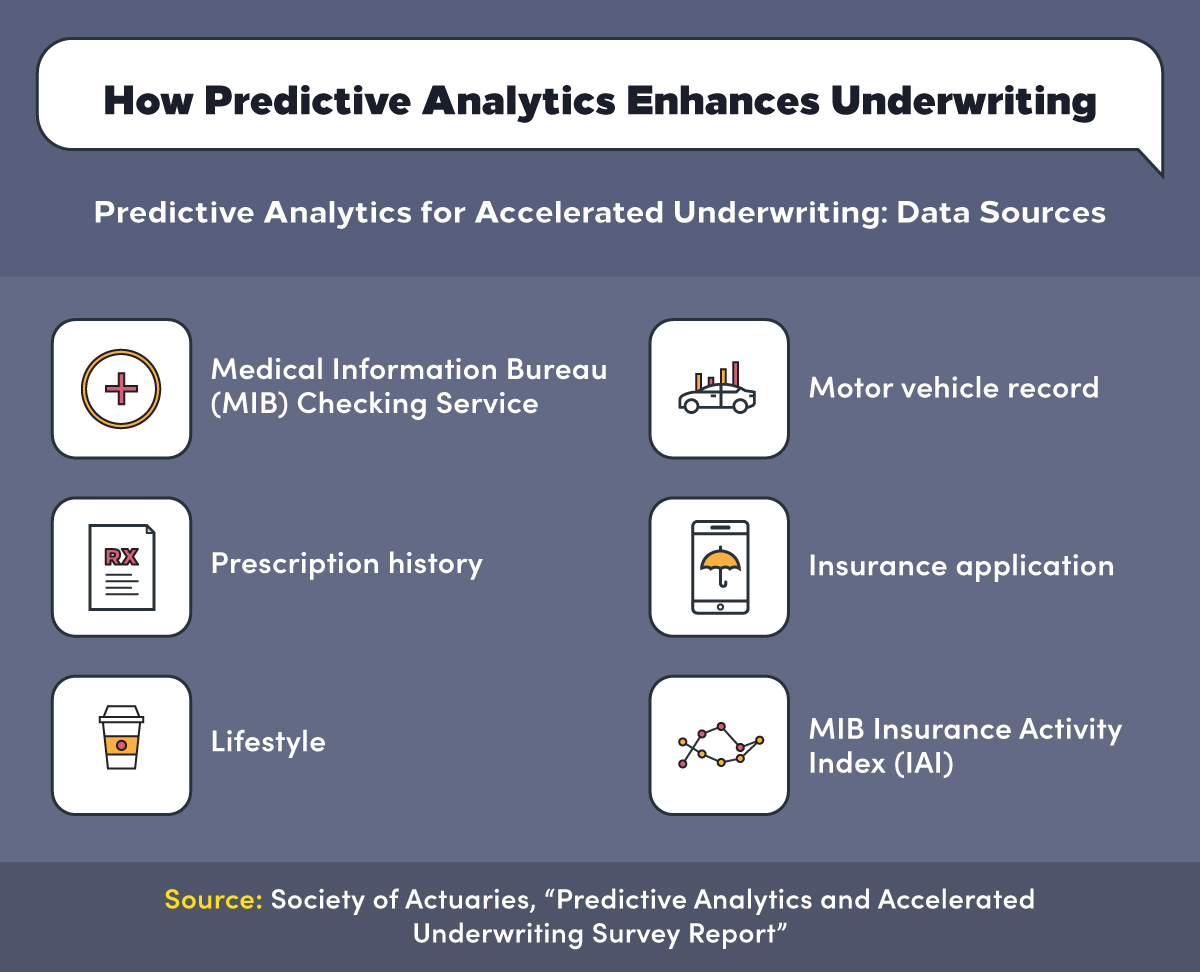
How Are Predictive Analytics Used in the Health Insurance Industry?
Recent years have seen a tremendous increase in the amount of electronic health data, including medical records and claims information. However, the health insurance industry hasn’t yet found ways to take advantage of this valuable resource. Predictive analytics in health insurance is seen as an opportunity for the industry to improve patient outcomes, enhance the efficiency of health claims processing, and reduce operating costs and patient premiums.
The challenge for health insurers in applying predictive analytics is to ensure high-quality data in addition to high-volume data, according to LexisNexis Risk Solutions. The article identifies three areas where health insurance firms benefit from the use of predictive analytics:
- Data-driven claims decisions
- Reduced operating expenses
- Improved profitability and expansion in new and existing markets
How Predictive Analytics Can Help Identify High-Risk Patients
According to the National Academy of Medicine, 5% of all patients account for nearly 50% of all healthcare spending. Predictive health analytics is seen as a way for healthcare providers to identify factors in their patients that are precursors to chronic illnesses and conditions. Similarly, health insurers are increasingly relying on predictive analytics to identify and engage high-risk patients in an effort to reduce inpatient admissions and emergency room visits.
An example of the application of predictive analytics to identify high-risk patients is the work done by the Health Care Transformation Task Force to develop care management programs directed at high-need, high-cost populations. The program uses qualitative information collected from physicians and patients and quantitative data from claims, demographic data, and other public sources.
How Predictive Analytics Can Reduce Healthcare Expenses
Healthcare legislation such as the Affordable Care Act of 2010 and the TRICARE program for current and former military members emphasize value-based insurance design (V-BID). V-BID is intended to increase the quality of healthcare and reduce healthcare costs via financial incentives promoting efficiency and consumer choice.
The V-BID approach has been adopted by many state governments and large insurers, such as Blue Cross and Blue Shield plans. Predictive analytics plays an important role in overcoming the obstacles to implementing value-based reimbursement models for healthcare providers and insurers. Dimensional Insight describes five ways data analytics support value-based care models:
- Identify gaps in care and at-risk patients most likely to experience a “crisis event.”
- Increase visibility into inappropriate use of high-cost health services.
- Address social determinants of health, such as food and housing security, patient health literacy, and transportation.
- Develop, test, and implement new workflows and care models.
- Enhance patient satisfaction and customer experiences.
Use of Predictive Analytics to Improve Claims Processing
Reinsurance provider Gen Re lists six ways predictive analytics is used by health insurers to optimize their claims processing operations:
- Allocation of resources/triage
- Reserving/settlement values
- Identification of potentially fraudulent claims
- Early warning of potentially high-value losses
- Expense management
- Trend analysis
A novel application of predictive analytics for health claims processing is in managing “outlier” claims that appear routine but run the risk of developing into high-value losses. An example is workers’ compensation claims that have the potential for long-term disability and permanency. Some seemingly minor claims, such as those involving soft tissue injury, may worsen over time and result in claims skyrocketing from the $8,000 to $10,000 range to the $200,000 to $300,000 range.
By applying predictive analytics to review historical claims data for similarities and other characteristics of such losses, insurers are able to identify “creeping catastrophic” (or creeping Cat) potential. Strategies and resources designed to minimize losses from such claims can then be applied early in the claims process to mitigate the potential for ballooning costs.
Resources for Predictive Analytics in Health Insurance
- Innovation Enterprise, How to Utilize Predictive Analytics in the Health Insurance Industry: This article discusses the use of social media analytics to strengthen relationships between physicians and patients.
- Managed Healthcare Executive, Making the Most of Predictive Analytics: This piece explains techniques used by healthcare providers and insurers to apply predictive analytics to improve care and control costs.
- Towards Data Science, 3 Tools for Healthcare Claims Data for Predictive Analytics: This article presents three types of predictive analytics models used in healthcare.
Predictive Analytics Tools for the Insurance Industry
Among the types of predictive analytics tools used by insurance companies are “what-if” modeling, claims prediction, and collection of external data from social media and other digital sources. These are among the popular applications for these and other analytics tools by the insurance industry.
Pricing and Product Optimization
- The Alteryx data analytics engine helps healthcare insurers and payers combine clinical, operational, and business data models to streamline processes and monitor costs. The product is used in call center support based on resource use and customer preferences, among other applications.
- Guidewire Live Analytics includes data models predesigned for specific insurance uses, including the Guidewire Claim Canvas that allows claims managers and catastrophe responders to geo-visualize claims and policy locations in combination with internal and external data.
Claims Prediction and Timely Resolution
- Lemonade runs insurance claims through an AI-based engine when they’re filed to detect fraud automatically, collect information from insured parties via chatbots, and approve claims on the spot when they meet prespecified criteria.
- Tractable is an AI-based system designed to assess car damage in real time, help insurers respond to disasters more quickly, and analyze the content of visual images. The company’s machine training techniques are optimized to handle uncertainty in datasets.
Predicting New Customer Risk
- Slice is an InsurTech firm that offers property and liability insurance on demand. The company’s AI-based Slice Mind service for insurers predicts risk by classifying business activities based on a website, a series of keywords, or a single natural-language sentence. The company also offers real-time cyber risk modeling, geographic distribution of risk, and a claims fraud detection service.
Fraud Detection and Policy Manipulation
- V2verify uses voice recognition to authenticate insured parties to healthcare providers, call centers, and other industries. In addition to enhancing customer experiences and improving access controls, it helps insurers ensure compliance with regulations and accommodate audits.
- ForMotiv’s Digital Polygraph predictive behavioral analytics models automatically identify and measure thousands of unique behavioral signals to establish a “digital body language” that predicts the intent of the people filing insurance claims and other online forms.
Dynamic Engagement of Customers
- ScoreData offers insurance companies real-time modeling designed to gain a better understanding of their customers’ changing behaviors and needs. The company provides risk-based granular pricing and advanced customer analytics, including customer lifetime value, retention and lapse analysis, social media analytics, and lead generation and conversion.
- Genesis Engage is a customer experience application that uses AI techniques to automate dialogues in self-service situations. The product uses “predictive routing” to determine the best agent for a specific customer and analyzes interactions with clients in real time to take advantage of the context of contacts and improve the customer experience.
The Future of Predictive Analytics Use in the Insurance Industry
Application of predictive analytics by the insurance industry is in its infancy. The future of predictive analytics for insurers promises to deliver more efficiency, higher profitability, and more engaged customers.
Extending Predictive Analytics Use to Other Types of Insurance
While current use of predictive analytics by insurers focuses on life, health, and vehicle coverage, other types of underwriting have proven more difficult to adapt to this and other AI-based technologies. In particular, the work of actuaries is challenging to convert to a machine-learning approach because the data models at the core of the analysis must be tweaked continually to account for variations in data, as NS Insurance explains.
One area in which predictive analytics is forecast to have a positive impact for insurers is in gaining insight into customer behavior and preferences. For example, by allowing insurers to create more detailed risk profiles of customers, the companies are able to offer affordably priced policies to high-risk clients, rather than having to deny them coverage outright.
Factors to Consider When Planning Investments in Predictive Analytics
Forbes lists the six key reasons why companies should invest in predictive analytics:
- Having a mature analytics process in place provides firms with a competitive advantage by identifying customers who are most likely to switch vendors and offering them incentives to remain.
- Companies are able to maximize the value of their internal data assets to enhance marketing operations and other business processes, especially in the area of customer engagement.
- The products identify areas at the corporate and department levels in which costs can be reduced, such as analyzing claims data to assist investigators working to prevent fraudulent claims.
- Predictive analytics provide companies with earlier notification of potential problems by analyzing customer and other data in near real time. For example, monitoring customer responses gives insurers insight into which innovations customers prefer.
- More classes of data can be included in the analyses that business decision-makers rely on, such as levels of price sensitivity among customers in specific demographics, and customer brand affinity.
- Predictive analytics enhance revenue growth over competitors that are slower to adopt predictive analytics. Research by Cisco Systems found that companies that have an enterprisewide analytics policy in place have average annual revenue growth greater than 7%.
Delivering the Benefits of Big Data to the Insurance Industry
The insurance industry is at the forefront of predictive analytics use to make operations more efficient and more profitable. However, the industry’s use of this and other AI-based technologies is in its infancy. While there will likely always be an important role for human actuaries and underwriters in insurance firms, their duties will continue to evolve as new technologies and data sources emerge.
At its heart, the insurance industry depends on the ability to predict outcomes and behavior changes. In the future, the only way to outperform the competition in this vital area is by taking advantage of predictive analytics tools and other AI products. As these tools become easier for business decision-makers to use, they’ll have a profound impact on all insurance providers and their customers.
Additional Sources
Daily Nation, “Big Data Has Quick Solutions for Insurance”
Duck Creek Technologies, “10 Ways Predictive Analytics in Insurance Will Shape the Industry in 2020”
Ethics and Insurance, “Why Predictive Analytics in Claims Is So Dangerous for Insurers”
InetSoft Technology, InetSoft Webinar: Predictive Analytics Examples in the Insurance Industry
PMA Companies, “Matching Predictive Analytics with Human Intelligence”
Toolbox, “How Can the Insurance Sector Benefit from Predictive Analytics?”
Virtusa, “Predictive Analytics in Insurance Claims”
Willis Towers Watson, “Predictive Analytics Speeds Innovation for Life Insurers”