The Analytics Bandwagon In 2017
The words “increase” and “continued evolution” sum up the direction Big Data will take in 2017. While the improvement of existing technology may not seem as exciting as new technology, the continued proliferation of cloud solutions, artificial intelligence, edge analytics, and data governance will make a big splash in the coming months and years.
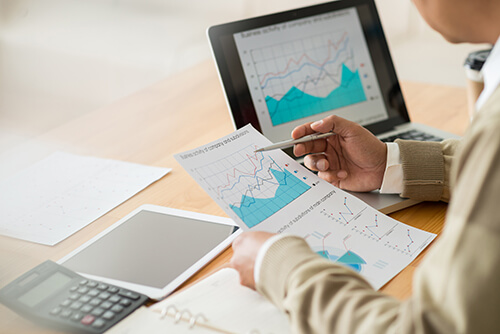
As the year continues, we will see increased value and insight from artificial intelligence (AI) and machine learning algorithms, which are now powerful enough to handle the Four Vs of Big Data: volume, velocity, variety, and veracity.
This year will also see more Internet of Things (IoT) sales, both among consumers and businesses, along with an increase in the use of distributed or “edge” analytics.
Better data-mining technologies and expanded analytics functionality are now highlighting the need for improved data governance to better organize data and adhere to privacy regulations and other data security restrictions.
Finally, 2017 will see a skyrocketing need for employees trained in up-to-date data science technologies and business intelligence operations. Students interested in a masters in business analytics or a masters in data analytics program may want to direct their studies toward an advanced degree in business data analytics.
AI And The Four Vs
The troublesome Four Vs of Big Data are responsible for the difficulties the business world has faced when attempting to analyze massive amounts of constantly growing data in a way that results in valuable, actionable insights.
The four Vs present obstacles to accurate and timely data analytics insights. The volume of data is incomprehensibly huge, which makes analytics difficult. The velocity, or the speed at which new data is added to already enormous data lakes, also presents a problem. The variety of data makes organization and pattern recognition difficult. And the veracity of data results in lots of useless data or “noise.”
The deep learning neural nets designed to alleviate these obstacles have had limited success at making a dent in the endless data lakes that have formed in the cloud.
Prior to 2017, one of the biggest issues facing AI had been that developers focused on specialized AI for specific verticals and business processes. General AI engines, on the other hand, will provide a versatile AI foundation that can be quickly and efficiently adapted to specific circumstances.
“More and more companies are applying artificial intelligence and deep learning into their applications, but a unified, standardized engine to facilitate this process has lagged behind. Today, to insert AI into robots, drones, self-driving cars, and other devices, each company needs to reinvent the wheel,” explains data science and machine learning consultant Daniel Gutierrez in his article, “Big Data Industry Predictions for 2017,” on InsideBigData.com, “In 2017, we will see the emergence of unified AI engines that will eliminate or greatly mitigate these inefficiencies and propel the formation of a mature AI tech supplier industry.”
Another recent development in AI involves information fusion (IF), which combines information into new, more concise and easily managed data sets. IF, when done correctly, reduces the degree of uncertainty attributed to analytics.
“The fusion of diverse information sources is a suitable solution for the operational needs of social Big Data analytics,” claims cloud computing researcher Ibrar Yaqoob, et al, in the 2016 article, “Information Fusion In Social Big Data: Foundations, State-Of-The-Art, Applications, Challenges, And Future Research Directions,” in the International Journal of Information Management. “And IF may allow companies to achieve their analytics goals more efficiently.”
IoT And “Edge” Analytics
Until 2017, the IoT was a largely over-hyped term applied mostly to smart watches and WiFi cameras. But the hype has passed into a reality that is only going to grow exponentially.
Today light bulbs, thermostats, vehicles, voice-activated home assistants, video doorbells, WiFi extenders, appliances, casting technology (e.g., Apple TV, Chromecast, Fire Stick), security systems, and even breathalyzers are all connected in some way.
Without local “edge” servers dedicated to processing and analyzing massive amounts of constantly streaming IoT data, the large centralized servers would soon be overworked.
Distributed analytics utilizes data, especially IoT data, near where it is generated and can return feedback to devices in a quicker, more efficient way. Some simpler analytics can even be done within each device’s local network without the use of any cloud server, local or centralized.
The newest trend in edge analytics is referred to as “fog” analytics and is bound to increase in popularity in 2017 and beyond.
“Fog analytics operates on the principle that processing should be done where it provides value,” says ARC Advisory Group Director of Research Mike Guilfoyle in his 2017 blog post, “Distributed Analytics Forecast: Partly Cloudy With A Chance Of Fog,” on Industrial-IoT.com. “Flexibility [of fog analytics] is the overriding functional requirement, as the analysis might occur at any layer of the operational footprint, extending to any horizontal or vertical point. Thus, a use case may use any mix of device-only, local, network layer, or enterprise analytics.”
Organization Through Governance
Data governance is becoming a prioritized issue for many companies, especially larger, global companies. Governance covers the effective and efficient organization of data, the quality of data, and data privacy.
“Data privacy is serious business and compliance with the new requirements can only be met with effective data governance providing transparency into data definitions, responsibilities, locations of storage, and data policies such as information access, retention, and destruction politics,” says data management solutions expert Jeff Shortis in his article, “Data Governance Trend And Predictions For 2017,” on Data3Sixty.com.
“The nature of these requirements and the negative effects of non-compliance will unite the goals of the chief data officer with those of the chief compliance officer and chief risk officer,” he says, “and finally provide data governance the teeth needed to truly impact the culture of organization.”
Maryville University’s MS In Business Data Analytics
In his InsideHigherEd.com article “Data Science Disconnect,” leadership and finance expert Paul Fain says of data science, “The demand touches many sectors, including finance, insurance, manufacturing, retail trade, and professional services. Data science and analytics-enabled jobs include human resources managers, business analysts, geographers, and market managers.”
Fain’s statement reflects the vast range of opportunities that await business analytics graduates. Maryville University’s Business Data Analytics program offers classes in everything from data mining and visualization to forecasting and predictive modeling, data governance, and warehousing.
Sources
Big Data Industry Predictions For 2017
Distributed Analytics Forecast: Partly Cloudy With A Chance Of Fog