What Is Tiny Machine Learning (TinyML)?
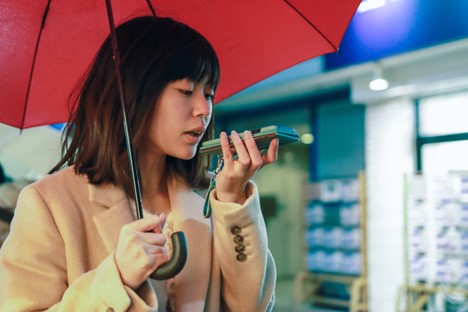
Machine learning is part of our everyday life. That’s because the concept exists at the core of a wide number of tech-driven innovations. Speech recognition devices use machine learning, as do chatbots that assist you on a website. Machine learning can also help detect fraud to keep your finances safe and enable computers to help connect you with online ads relevant to your interests. It’s become increasingly difficult to go through a day without having a machine learning-based interaction.
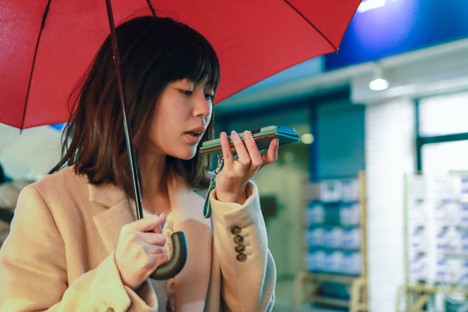
However, machine learning hasn’t just arrived — it’s evolving. A prime example is the emergence of tiny machine learning. Also known as TinyML, this concept arguably represents the future of machine learning, as it makes it possible to expand the reach and possibilities of machine learning to a wider list of devices. What is TinyML exactly, and why can it make such a potentially profound impact on our everyday lives?
What Is TinyML?
TinyML makes it possible to bring machine learning capacities to low-power and battery-operated machines — devices that otherwise wouldn’t have the capacity to sufficiently handle full machine learning systems. These devices typically operate on less than one megawatt of power; this allows them to operate for long periods of time without needing a replacement for functional elements, such as batteries.
Unlike the typical machine learning application, TinyML doesn’t rely on elements such as microprocessors and graphics processing units (GPUs). Rather, it functions on limited memory, such as a few kilobytes’ worth of RAM, and minuscule amounts of megahertz. Despite its limited capacity, TinyML uses the same machine learning and deep learning tools found in larger machine learning systems. These include sensors, algorithms, and data analytics tools that process information and dispense accurate results. Because of size, these functions are scaled through quantization: a process that allows for the reduction of bit size so that it can take up less memory and use less power.
TinyML’s functionality and design make it an essential component of edge computing devices. These devices make it possible for data processing and analysis to occur closer to the data’s origin point than a data center or cloud network. Ultimately, this creates a one-two punch that allows for greater analytical efficiency, making these devices significantly more useful from both a user and an organizational standpoint.
TinyML Functionality and Use
The applications that TinyML brings to the table are of interest to many computing professionals, transcending the differences that may exist between professions like machine learning engineer and data scientist. To fully understand how TinyML functions, diving into its ecosystem is important. This system builds on a series of microcontrollers, software, and deep-learning frameworks that can forego the need of the cloud, the internet, or a data center to run machine learning models. A popular example of this ecosystem is Google’s TensorFlow Lite: an open-source ecosystem designed to function across a broad range of supported platforms.
The ecosystem provides the backbone for an increasingly wide range of devices that use artificial intelligence-driven concepts, such as wake-word detection models, gesture recognition, and machine monitoring. A prime example of TinyML’s application is the wake-word detection model found in smartphones that only needs 14 kilobytes running on a demand-side platform (DSP) to fully and effectively respond to a basic voice command, such as “OK, Google.”
As its use continues to evolve, TinyML has an efficiency that can lend itself to several applications over a wide range of industries. TinyML can be beneficial in the agricultural technology industry, as it can boost the efficiency of crop management and livestock tracking. In the retail sector, TinyML can help store managers track inventory with greater efficiency and help them gain sharper insight into consumer behaviors. TinyML can also be useful in the healthcare sector, as it can be used to improve vital signs monitoring, track the progress of degenerative health conditions, and improve patient privacy.
TinyML: Benefits and Challenges
Using TinyML technology can yield several benefits. Because they’re specifically designed to work with such low-power devices, TinyML systems themselves don’t consume much energy. This also allows them to be cheaper than more elaborate machine learning systems with more moving parts. TinyML systems can also potentially boost the security of data because all their data and subsequent analysis happens close to the data’s origin point and doesn’t have to be uploaded to the cloud.
On the other hand, a few challenges to TinyML use need consideration. For example:
- The lack of homogeneous consistency between TinyML implementations, making it difficult to share or translate insights across multiple TinyML ecosystems
- The lack of benchmarking tools and procedures regarding TinyML systems, making it tougher to gain a comprehensive understanding of system effectiveness or efficiency
- The limited number of acceptable models to use
Despite the hurdles, however, the benefits associated with TinyML create a potential that can’t be ignored. As the technology continues to evolve, it’s not a stretch to think that these hurdles will one day be cleared.
Bring Tomorrow’s Tech to Today’s Landscape
TinyML’s ability to shrink big AI and machine learning concepts to work with smaller and handheld devices is nothing short of revolutionary. For individuals in the technology and analytical technology sectors, TinyML represents a glimpse into a thrilling future, one that’s driven by greater efficiency, security, and opportunity. It’s a game changer that, given its current presence on the market, is already changing the game.
Maryville University’s online Master of Science in Artificial Intelligence degree and its Artificial Intelligence certificate can provide a great entry point into this dynamic world. Each is designed to help you cultivate the knowledge and skills you need to not only fully understand the intricacies of this burgeoning concept, but also lead the field in innovation and discovery. Learn how we can help you become a leader who moves technology further into the future.
Recommended Reading
Building Skills for the Future of Machine Learning
The Future of Big Data in Business: How Data Analytics Provides Insight
Sources:
All About Circuits, “What Is TinyML?”
Artificial Intelligence Board of America, TinyML: The Future of Machine Learning
IBM, What Is Machine Learning?
TechTarget, “Why TinyML Use Cases Are Taking Off”
TensorFlow, TensorFlow Lite for Microcontrollers
TinyML Foundation, About TinyML Foundation
TinyML Foundation, TinyML Talks: A Practical Guide to Neural Network Quantization