4 Benefits of Data Analytics in Healthcare
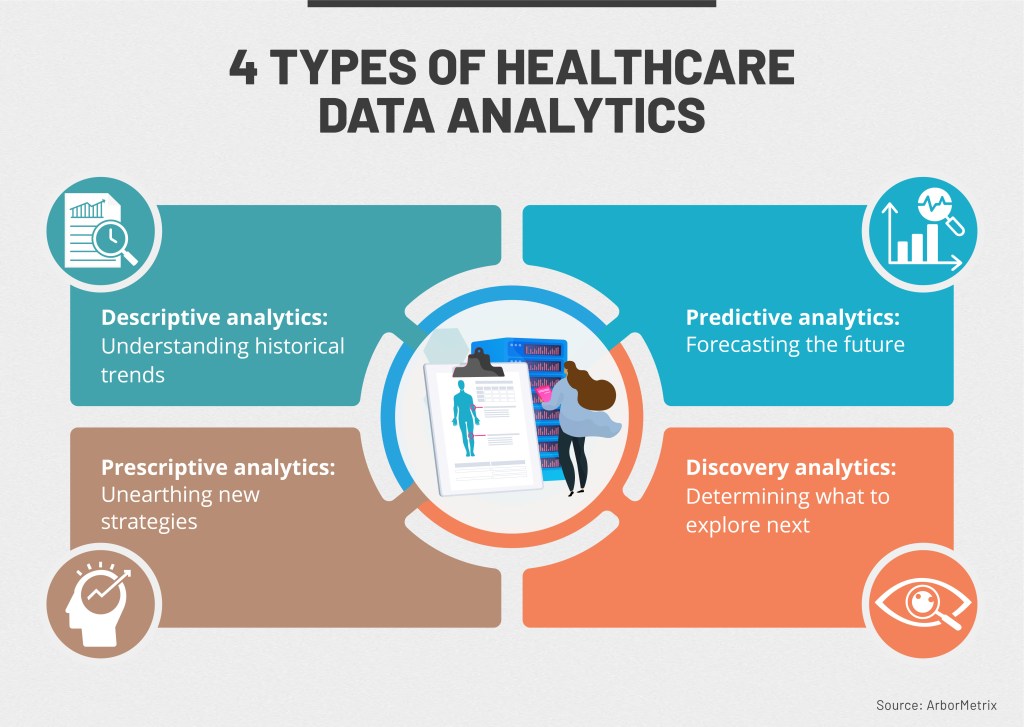
Tables of Contents
The world is being inundated with data:
- The amount of data in the world grew by 5,000% between 2010 and 2020.
- The total amount of data generated each year doubles about every three years and is estimated to reach 79 zettabytes in 2021.
- Netflix’s use of predictive analytics has allowed the streaming service to influence 80% of the movies and TV shows that its 100 million subscribers selected.
Like many natural resources, raw data has limited value until it’s refined to make it suitable for people to use. Converting data into a valuable commodity requires advanced analytics techniques. Data analytics is the process of analyzing data in its many structured and unstructured forms through various methods: The goal is to spot trends and unlock insights that weren’t otherwise evident.
All industries are poised to benefit from the use of data analytics to convert this valuable raw material into business intelligence, but no sector will feel the impact of this technology more than the ever-evolving healthcare industry. As Grand View Research reports, the global market for data analytics in healthcare was valued at $26 billion in 2019 and is expected to increase at an annual growth rate of 7.5% from 2020 to 2027.
In areas as diverse as cancer treatment, drug discovery, and disease prediction, data analytics will transform the provision of healthcare services. The application of advanced analytics techniques and the continuing geometric growth in the amount of data available to analyze will affect nearly all areas of healthcare, including the accuracy of insurance rates, the automation of administrative processes, and the use of artificial intelligence (AI) in diagnostics.
What is data analytics in healthcare?
The healthcare industry generates a tremendous amount of data but struggles to convert that data into insights that improve patient outcomes and operational efficiencies. Data analytics in healthcare is intended to help providers overcome obstacles to the widespread application of data-derived intelligence:
- Making healthcare data easier to share among colleagues and external partners, and easier to visualize for public consumption
- Providing accurate data-driven forecasts in real time to allow healthcare providers to respond more quickly to changing healthcare markets and environments
- Enhancing data collaboration and innovation among healthcare organizations to convert analytics-ready data into business-ready information by automating low-impact data management tasks
The tools used in analytics fall into three general categories:
- Software that acquires the data from sources that include patient surveys, case files, and machine-to-machine data transfers
- Programs that clean, validate, and analyze the data in response to a specific research question
- Software that builds on the results of the analysis to suggest various actions to achieve specific healthcare goals
Along with collecting, analyzing, and interpreting data, analytics software must secure the data and the analysis results while ensuring that the healthcare professionals who’ll benefit from the insights have ready access to the information in a form that they can easily use in their work.
How data analytics is used in healthcare settings
Data analytics in healthcare can be applied to every aspect of patient care and operations management. The analyses investigate methods of improving the provision of clinical care, enhancing disease prevention, and measuring the effectiveness of various treatment options.
The ability of data analytics to convert raw healthcare data into actionable intelligence is expected to have the greatest impact on these areas of healthcare:
- Research and prediction of disease
- Automation of hospital administrative processes
- Early detection of disease
- Prevention of unnecessary doctor’s visits
- Discovery of new drugs
- More accurate calculation of health insurance rates
- More effective sharing of patient data
- Personalization of patient care
An example of the use of data analytics in a clinical setting is the library of molecular and clinical data that Tempus is creating to give healthcare professionals a clearer clinical context for a cancer patient’s unique case. The data is collected from lab reports, clinical notes, radiology and pathology images, and accelerated cancer research. The project’s goal is to provide oncologists with tools to help them personalize their patients’ treatments.
Public health officials are using data analytics to improve the prevention of disease and identify members of the population who are most at risk. For example, Linguamatics has created an analytics engine that uses natural language processing to analyze the unstructured patient data in health records to identify lifestyle factors that its predictive models associate with high-risk patients.
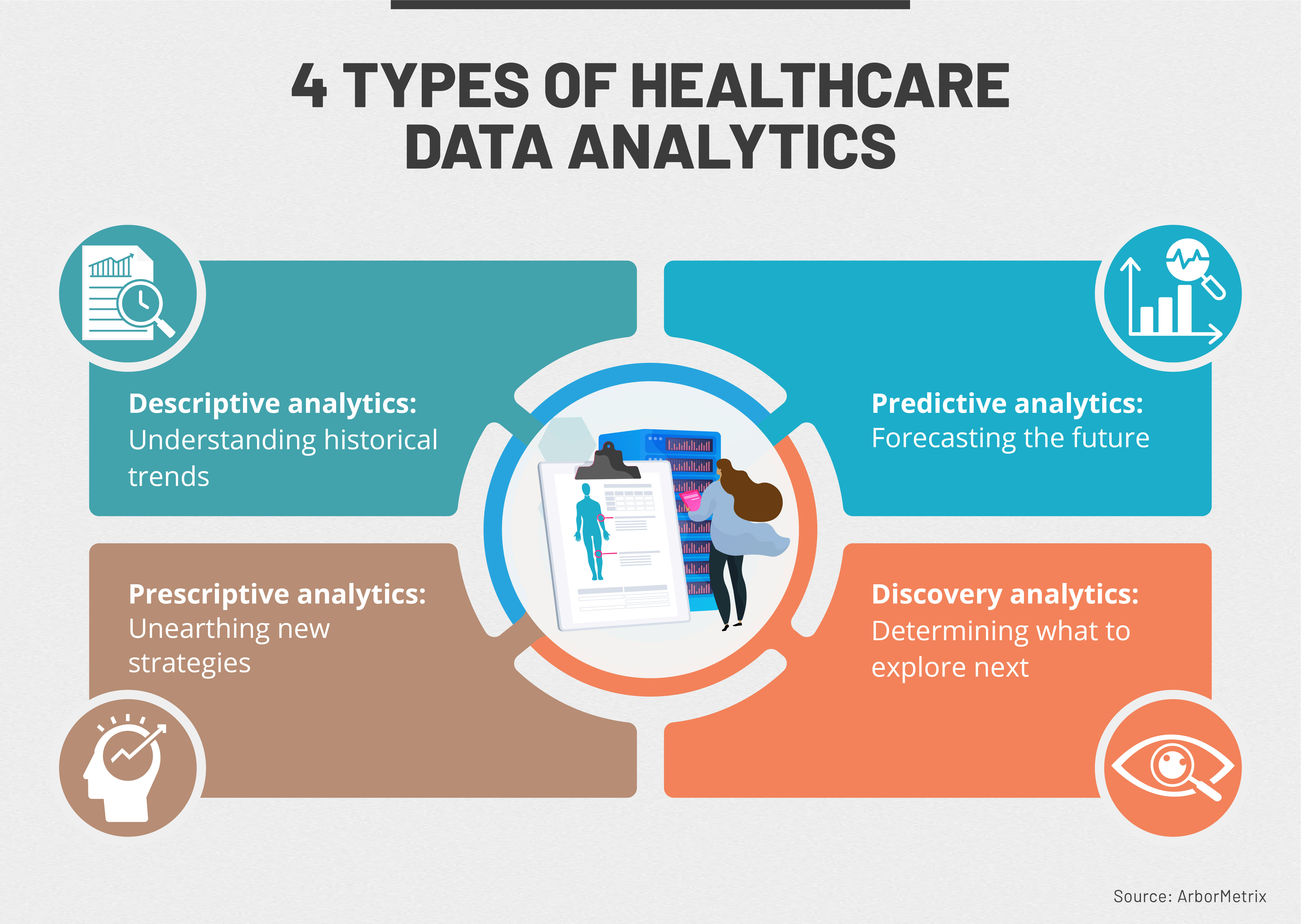
According to ArborMetrix, descriptive analytics are used to understand historical trends; predictive analytics to forecast the future; prescriptive analytics to unearth new strategies; and discovery analytics to determine what to explore next.
Who performs data analytics for healthcare applications?
The people responsible for performing data analytics in various healthcare settings include researchers, physicians, hospital administrators, and insurance industry executives. Healthcare professionals work in conjunction with people trained in the use of advanced data analytics techniques, but they also may work directly with new analytics tools that feature graphical interfaces for people without a background in analytics.
These three groups of healthcare providers are the most common users of data analytics in healthcare:
- Clinical practitioners. Data analytics in clinical settings attempts to reduce patient wait times via improved scheduling and staffing, give patients more options when scheduling appointments and receiving treatment, and reduce readmission rates by using population health data to predict which patients are at greatest risk.
- Healthcare payers. Insurance firms use data analytics to confirm that they comply with ever-changing regulations; analyze claims and prescriptions to target prevalent health maladies; and compare pricing data with quality metrics to identify high-value, low-cost health providers. Insurance firms also use predictive analytics to spot the potential for fraudulent claims and notify providers of at-risk claims.
- Population health managers. Public health professionals increasingly emphasize prediction and prevention over response and treatment. Predictive analytics is used to identify patients at highest risk of chronic illness at the early stages of the disease. Analysis of lab testing, claims data, data that patients themselves generate, and various social factors reduces the risk of long-term illness, which lowers overall healthcare costs and improves patient outcomes.
What are the benefits of data analytics in healthcare?
Many different types and forms of data are combined when analytics techniques are applied in healthcare settings, medical research, and public health departments. Health-related data sources include electronic health records (EHRs), genomics and post-genomics, bioinformatics, medical imaging, sensor informatics, medical informatics, and health informatics. The analysis entails many discrete steps:
- Integrate heterogeneous data types.
- Ensure the quality of the data upon reception and throughout the analysis.
- Create data models.
- Interpret the results of the analysis.
- Validate the analysis results.
The benefits of data analytics in healthcare are realized in seven distinct areas:
- Support clinical treatment decisions from physicians and other health professionals.
- Improve the accuracy and speed of identifying patients at highest risk of disease.
- Provide greater detail in the EHRs of individual patients.
- Make the provision of healthcare more efficient, which reduces costs.
- Promote preventive measures by giving patients greater insight into their health and treatment goals.
- Integrate data from consumer fitness devices and other patient-provided sources of health data.
- Deliver real-time alerts to healthcare providers by analyzing health data at the collection point.
How data analytics is used to advance medical research
The use of data analytics in medical research promises to improve the ability of public health organizations to predict the outbreak of disease, improve the prevention of disease, enhance the quality of life, and extend the lifespan. Research that data analytics supports is addressing the most pressing health problems that global populations face:
- Data scientists from Blue Cross Blue Shield and analytics firm Fuzzy Logix have identified 742 risk factors that accurately predict when a person is at risk of abusing opioids.
- The Cancer Moonshot initiative that former President Barack Obama initiated in his second term relies on advanced data analytics techniques to speed the discovery of cancer cures by identifying the trends and treatments used worldwide that have the highest success rate.
- The research collaboration firm OptumLabs has created a database of more than 30 million EHRs that doctors can access to support their treatment decisions. The database has proven to be especially helpful in treating patients who have complex medical histories or who suffer from multiple conditions.
- AI-based analytics promises to allow radiologists to “read” images via algorithms that can more accurately identify patterns that indicate a particular diagnosis.
- Kaiser Permanente worked with the Mental Health Research Network to analyze EHRs and the results of a standard depression questionnaire to identify with great accuracy patients at highest risk of attempting suicide.
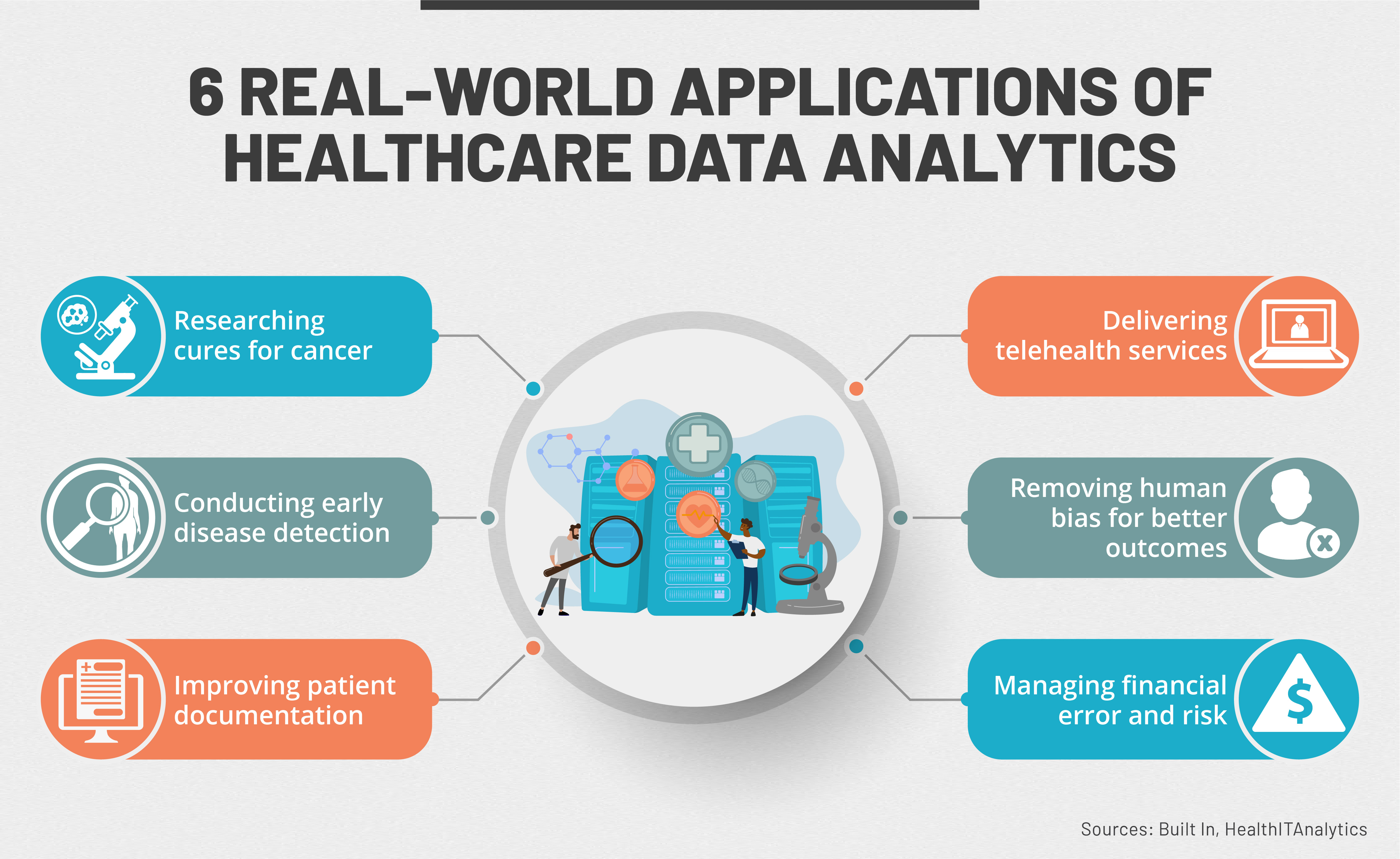
According to Built In and HealthITAnalytics, data analytics help research cures for cancer, conduct early disease detection, improve patient documentation, deliver telehealth services, remove human bias for better outcomes, and manage financial error and risk.
Benefit #1: Analyzing clinical data to improve medical research
Data analytics techniques are being applied to improve research efforts in many health-related areas by gathering and analyzing clinical data from various sources. Among the most useful sources of clinical information are EHRs, electronic medical records, personal health records, and public health records.
- EHRs combine a patient’s X-rays and other medical images, diagnoses, treatment plans, allergies, and test results in standard digital formats. This makes the information easy to share but introduces privacy and regulatory compliance requirements that limit how the data may be used.
- Electronic medical records are similar to EHRs but include only information from the patient’s paper charts created in medical offices, clinics, and hospitals. They are used primarily for diagnosis and treatment; their main value is in tracking a patient’s healthcare over years of visits and screenings.
- Personal health records maintain a history of the patient’s health treatment that the patient keeps rather than healthcare providers. The records are intended to assist in the patient’s own health management and don’t legally replace the medical records that healthcare providers maintain.
- Public health records are among the most promising sources of health data for medical research. For example, the National Cancer Institute’s Cancer Research Data Commons (CRDC) serves as a cloud-based data science platform that links data analytics tools with data repositories storing genomic, proteomic, comparative oncology, imaging, and other data types.
Other sources of clinical data are patient and disease repositories, such as the National Program of Cancer Registries, and clinical trial data from sources that include the GlaxoSmithKline Study Register.
Data analytics benefits medical researchers in three primary ways:
- The results help researchers identify approaches to improve the efficiency of clinical processes and other healthcare operations.
- The research leads to more accurate diagnosis and treatment by personalizing healthcare provision.
- Cohort studies provide medical researchers with new insight into the causes of disease by linking risk factors with health outcomes.
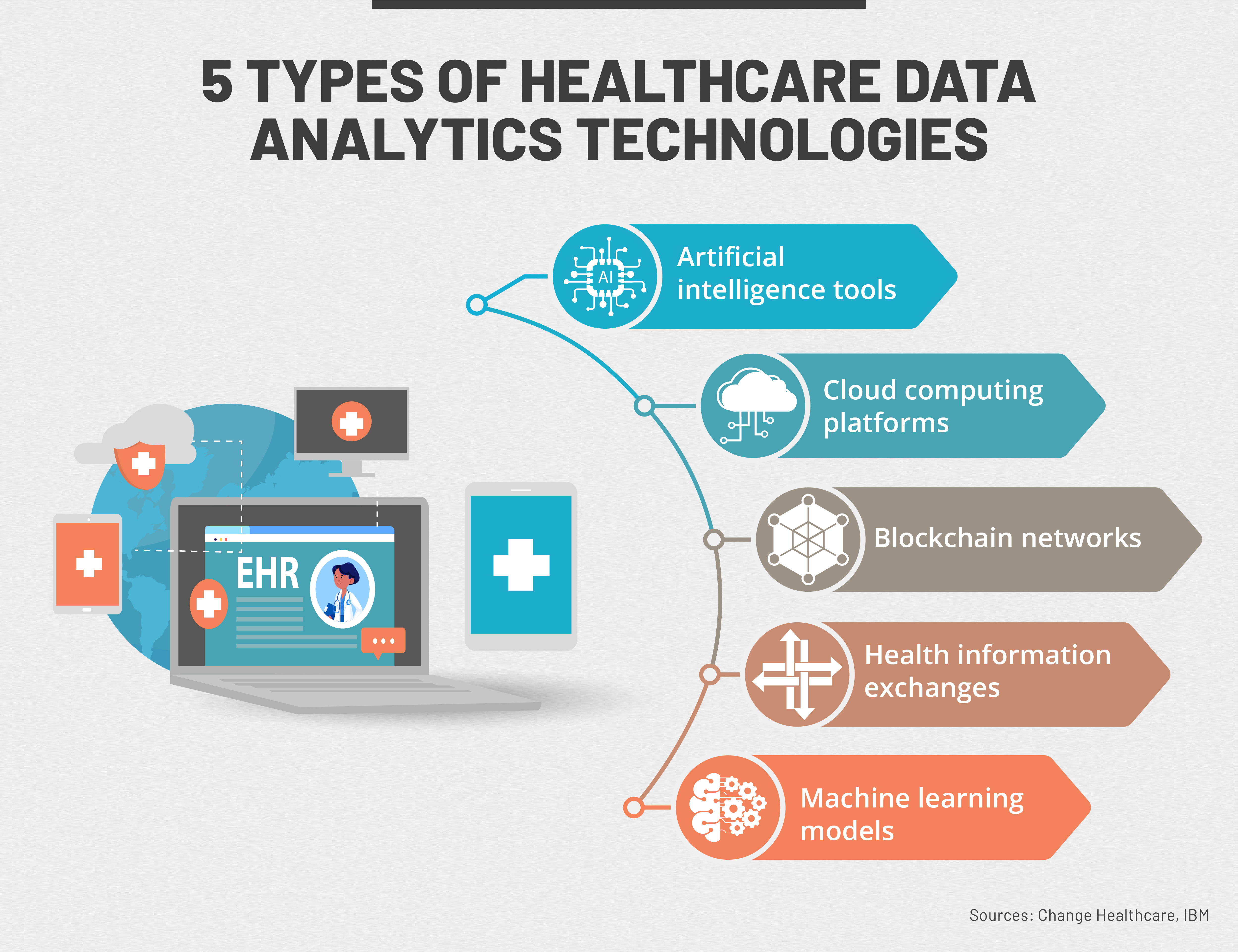
According to Change Healthcare and IBM, healthcare providers use these five data analytics technologies: artificial intelligence tools, cloud computing platforms, blockchain networks, health information exchanges, and machine learning models.
Benefit #2: Using patient data to improve health outcomes
The goal of quality improvement in healthcare settings is to treat patients safely and effectively while minimizing the trauma associated with their treatment. To achieve this goal, healthcare providers collect and analyze patient data, increasingly in real time, to gain a clearer understanding of today’s complex healthcare environments; to develop and apply a systematic approach to improve patient outcomes; and to continuously develop, test, and implement enhancements to healthcare processes.
By analyzing patient data, healthcare providers can lower readmission rates, reduce errors, and better identify at-risk populations. The types of patient data used in these analyses include blood sugar level, temperature, blood test results, and the patient’s own wishes for care. The primary factors that influence the quality of care patients receive are:
- The health outcomes that patients expect and that matter most to them
- How the processes that healthcare providers use impact patients’ desired outcomes
- How the resources, equipment, regulations, and other aspects of healthcare infrastructure affect the quality of care that patients receive
Healthcare professionals face many challenges in gaining timely access to patient data that can help them improve the quality of the services they provide. One way to avoid being overwhelmed with patient data is to focus on a handful of key performance measures and collect only as much data as needed to track those measures. This requires making qualitative assessments of the patient data and ensuring that the measures are accessible to providers and trackable over time to mark improvement gains.
Patient-reported outcome measures (PROMs) differ from process measures of improvement, such as avoidable readmissions, hospital-acquired infections, and mortality, by focusing on the outcomes that matter most to patients, namely:
- Recovery from acute illness
- Living well while managing a chronic condition
- Maintaining dignity at the end of life
Data collected from patients to assess their care includes how they feel about their overall health, how well they can complete common activities, their mood, their energy level, and how much pain they’re experiencing. Use of PROMs in healthcare settings is slowed by the difficulty in collecting this data from patients because of the time and effort required. Healthcare providers struggle to link patient-reported outcomes to specific treatments because of the many social and demographic factors that influence patient outcomes.
To make patient data easier to collect and analyze, the National Institutes of Health has created the Patient-Reported Outcomes Measurement Information System (PROMIS) that’s designed to evaluate and monitor the physical, mental, and social health of adults and children. Healthcare providers can view a demo of the PROMIS Computer Adaptive Tests (CATs) in various categories, including anger, anxiety, depression, fatigue, pain behavior, pain interference, physical function, and satisfaction with social activities and social roles.
Benefit #3: Gaining operational insights from healthcare provider data
The work processes and organization structures of healthcare providers directly impact the quality of care patients receive and the likelihood of positive patient outcomes. Measuring the effect of process and organization changes on outcomes and operational efficiencies requires the ability to collect and analyze healthcare provider data related to these processes and structures in complex healthcare environments.
Improving the quality and efficiency of healthcare starts with an organization-wide assessment of current conditions combined with an alignment of the institution’s strategies and goals for improvement. The operational areas most likely to benefit from data analytics include employee scheduling, charting, emergency preparation, administration, financial management, and compliance.
Among the approaches that healthcare providers have taken to enhance their operational efficiencies while maintaining a high standard of care are:
- Waste reduction. The annual cost of recoverable waste in the U.S. healthcare industry is estimated at $1 trillion. Much of the waste is due to variations in clinical practices, inappropriate care, preventable care-related injury and death, and failure to follow proven procedures.
- Cost-effective use of technology. Adopting analytics and other advanced technologies is expensive and time consuming. A framework for cost-effectively implementing data analytics focuses on measuring, understanding, and improving those operations with the most promise for improved outcomes.
- Increasing hospital capacity. A less-expensive alternative to building new healthcare capacity is to apply analytics techniques to better manage demand for hospital beds and other healthcare resources.
- Improved project management. As healthcare organizations adopt the Institute for Healthcare Improvement’s Triple Aim framework for enhanced patient outcomes and operational efficiency, project management becomes an integral part of cost control, risk management, and successful process improvement.
- Sustaining outcome improvements. Efforts to implement data-driven enhancements to healthcare operations often underestimate the resources needed to sustain the improvements. To ensure the long-term success of analytics efforts, organizations should engage all stakeholders at every stage of the process.
Benefit #4: Improved staffing through health business management analytics
Data analytics is used in health business management to identify staffing issues and recruit, hire, train, and retain healthcare workers. Healthcare providers have to balance the need to contain costs and improve efficiency and patient outcomes with the growing shortage of qualified medical professionals. Labor costs account for about 60% of all hospital budgets, and the increasing demand for healthcare staff is expected to drive salaries and wages even higher in the future.
Data analytics is being applied to manage labor costs in healthcare settings while simultaneously improving the quality of care patients receive and the efficiency of service provision. For example, Hawaii Pacific Health (HPH), one of the largest healthcare systems in Hawaii, was able to save $2.2 million over 16 months while maintaining high-quality outcomes by adopting a data-driven approach to labor management.
HPH’s paper-based management system was replaced with computer-generated visual representations of labor utilization that combined real-time views of staffing with graphical representations of staff levels. This transition gave managers greater insight into the productivity of individual workers and teams and the cost-effectiveness of staffing decisions, allowing the hospital to adjust staffing ratios in a way that reduced its labor costs without affecting the quality of care provided or patient outcomes.
The automated labor management system cut the time managers spend on scheduling from four hours to only 15 minutes. The system also allows hospital staff to find answers to staffing and other questions in an instant rather than the two weeks required when using the paper-based approach to scheduling. Hundreds of workers at the hospital use the automated solution to manage their schedules and other employment-related resources.
Applying health data to improve patient outcomes
The impact of data analytics in healthcare has already made a substantial difference in the ability of healthcare providers to offer patients high-quality care in an efficient, cost-effective manner. However, the role of data analytics in improving patient outcomes and healthcare processes continues to grow and expand as more types of data become available and new tools are developed that make the results of the analytics clear and easy for healthcare professionals to access.
Realizing the potential of data analytics to transform the healthcare industry begins by understanding how the technology can be applied to address healthcare providers’ challenges, including staff recruitment and utilization, operational efficiencies, and enhanced patient experiences. Patient-centered healthcare depends on knowing what patients want and need. Data analytics holds the key to unlocking this vital information.
Infographic Sources
ArborMetrix, “What Is Healthcare Analytics and Why Is It Important?”
Change Healthcare, “Healthcare Data & Analytics Solutions”
Health IT Analytics, “4 Emerging Strategies to Advance Big Data Analytics in Healthcare”